Unlocking the Power of Image Annotation Tools for Machine Learning
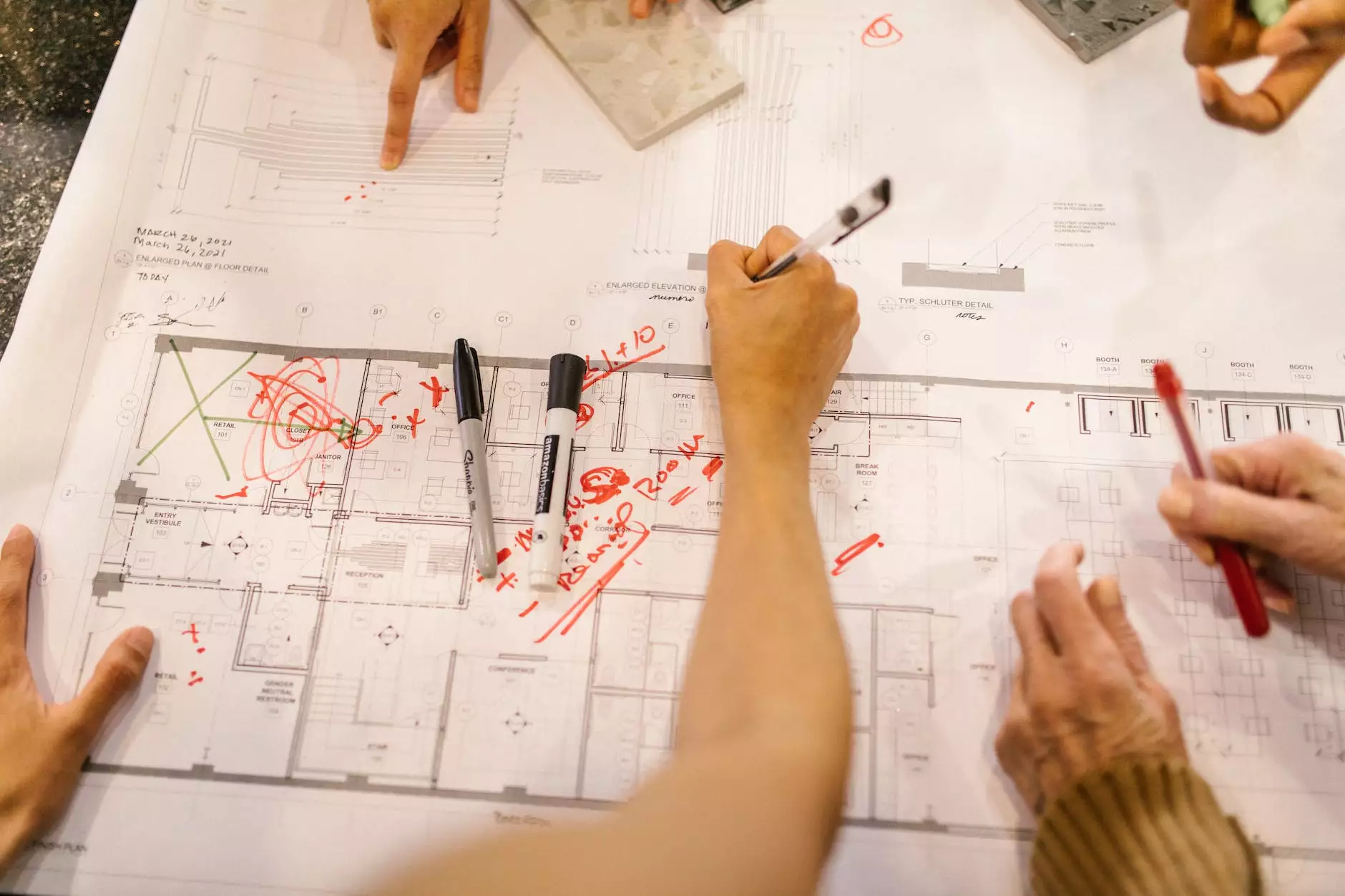
In the vast and evolving landscape of artificial intelligence, image annotation tools for machine learning serve as a critical bridge between raw data and actionable insights. The growth of AI applications has increased the demand for high-quality, annotated datasets. This article explores the significance of these tools, highlighting their myriad benefits, features, and best practices to ensure successful implementation in your business strategy.
Understanding Image Annotation
Image annotation is the process of labeling images to provide context for machine learning algorithms. These annotations can take various forms, including:
- Bounding boxes: Rectangles drawn around objects to identify their location.
- Segmentation: Dividing an image into different segments or boundaries for more granular identification.
- Key point annotation: Indicating key points on objects, such as facial features in images of people.
- Text annotation: Tagging images with descriptive texts to help machines understand the nuances of visual data.
Each annotation method plays a vital role in training machine learning models, allowing them to learn patterns and make predictions based on visual input.
The Importance of Image Annotation Tools for Machine Learning
When it comes to training machine learning models, having accurately annotated images is of utmost importance. Here are several reasons why image annotation tools for machine learning are essential:
1. Enhancing Data Quality
Quality training data is paramount for effective machine learning. Image annotation tools ensure that every image is meticulously labeled, enhancing the data's reliability. Higher quality data leads to improved model performance and more precise predictions.
2. Streamlining Workflows
Manual image annotation can be time-consuming and error-prone. By utilizing advanced annotation tools, businesses can automate parts of the process, reducing human error and increasing efficiency. This allows teams to focus on higher-level tasks rather than repetitive data labeling.
3. Scalability
As the demand for AI applications grows, so does the need for vast amounts of labeled data. Image annotation tools provide scalable solutions that allow businesses to annotate thousands of images quickly, ensuring that they keep pace with their growth and the evolving market needs.
4. Supporting Diverse Use Cases
From healthcare to autonomous vehicles, annotated images are used across a multitude of industries. Whether you are training models to detect tumors in radiology images or teaching drones to identify obstacles, the flexibility of image annotation tools supports a wide array of applications.
Key Features of High-Quality Image Annotation Tools
Choosing the right image annotation tool for machine learning requires understanding the key features that distinguish the best from the rest. Here are some critical features to look for:
User-friendly Interface
The best image annotation tools provide an intuitive interface that simplifies the annotation process, making it easy for both technical and non-technical team members to contribute.
Customization Options
Different projects require different annotation approaches. An effective tool should allow users to customize labeling options, whether through bounding boxes, segmentation, or other methods.
Collaboration Features
In today’s working environment, collaboration is crucial. The best annotation tools enable multiple users to work simultaneously, facilitating teamwork and improving productivity.
Integration Capabilities
For seamless operations, image annotation tools should integrate easily with other platforms such as machine learning frameworks, data storage solutions, and project management tools.
Quality Assurance Mechanisms
To ensure that annotations meet the required standards, quality assurance features allow teams to review and approve annotations, minimizing errors before training models.
Best Practices for Implementing Image Annotation Tools
To fully leverage the potential of image annotation tools for machine learning, businesses should adhere to certain best practices:
1. Define Clear Objectives
Before embarking on the annotation process, clearly define the objectives of your project. What are the specific outcomes you hope to achieve with your machine learning model? Having a clear direction will help guide the annotation process.
2. Choose the Right Tool
Select an annotation tool that aligns with your project needs and team capabilities. Consider features like scalability, user experience, and integration options to make the best choice.
3. Train Your Team
Providing adequate training for your team members on how to use the chosen tool effectively is vital. A well-trained team can maximize the tool's capabilities, leading to better results.
4. Regularly Review Annotations
Incorporate regular reviews of the annotations to ensure quality control. Feedback loops will help in refining the annotation process and improving the accuracy of your datasets.
5. Utilize Automation Wisely
While automation can significantly enhance efficiency, it's essential to identify tasks that still require human expertise. A balanced approach between automated and manual annotation can yield the best results.
The Future of Image Annotation in Machine Learning
As artificial intelligence continues to evolve, so too will the tools and strategies used for image annotation. Here are some trends shaping the future:
1. Artificial Intelligence in Annotation
Interestingly, AI itself is becoming integral to image annotation processes. Innovative tools are emerging that use machine learning to assist in the annotation process, automatically suggesting labels and speeding up the workflow.
2. Increased Focus on Quality
With the rising importance of ethical AI, there's a growing focus on ensuring the quality of annotated data. Future tools will likely incorporate advanced quality control mechanisms to enhance data reliability.
3. Diversification of Annotation Types
As AI applications become more complex, the demand for diverse annotation types will increase. Expect to see tools that support not just static images but also video annotations and real-time data labeling.
4. Global Collaboration
The need for high-quality annotated data is universal. As such, collaborative annotation tools that allow teams from around the world to contribute will become increasingly valuable.
Conclusion
In the realm of machine learning, the significance of image annotation tools for machine learning cannot be overstated. They are a foundational element that enhances data quality, improves workflow efficiency, and supports diverse applications across numerous industries. As technology progresses, embracing the right annotation tools and following best practices will empower businesses to harness the full potential of their data, paving the way for innovative AI solutions.
At Keylabs.ai, we provide cutting-edge data annotation tools and a versatile data annotation platform designed to meet the demands of modern AI projects. By partnering with us, you can ensure that your machine learning models are built on a solid foundation of high-quality, accurately annotated data.