Transforming Business Operations with Labeling Tool Machine Learning
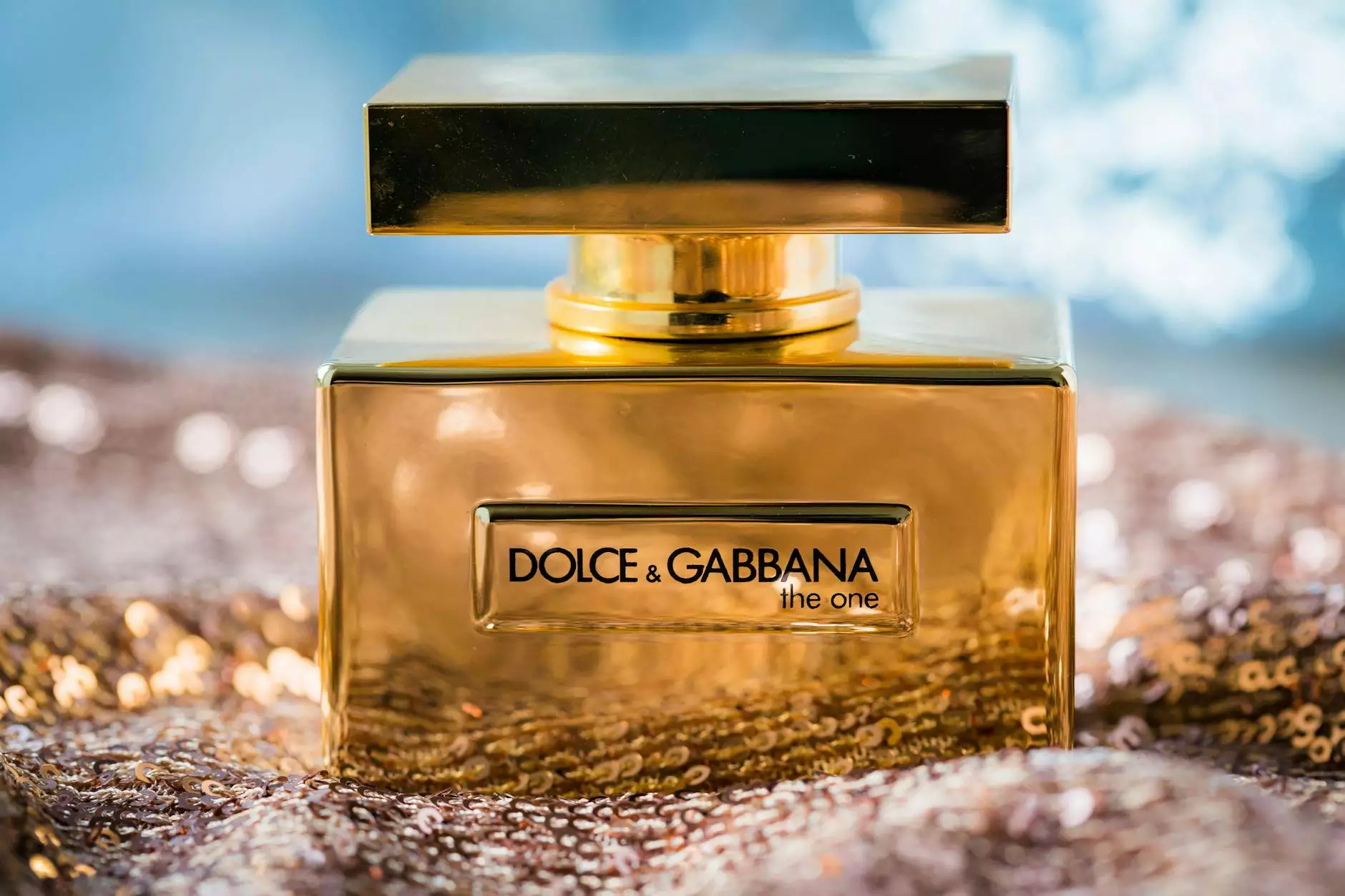
In today's digital landscape, businesses are leveraging advanced technologies to streamline processes, enhance productivity, and improve decision-making. One pivotal technology in this evolution is labeling tool machine learning. These tools are transforming how data is managed, annotated, and utilized across various sectors. In this comprehensive article, we will delve deep into the significance of labeling tools in machine learning, particularly in the context of the data annotation tool and platform domains offered by KeyLabs AI.
Understanding Machine Learning and Data Annotation
Machine learning (ML) is a subset of artificial intelligence (AI) that enables systems to learn and improve from experience without being explicitly programmed. Central to the success of machine learning algorithms is the quality and volume of data they are trained on. Thus, data annotation becomes a critical step in leveraging machine learning effectively.
Data annotation involves labeling data to provide context and meaning to unstructured data sets. This step is essential for training ML models as it helps algorithms make accurate predictions and classifications. For instance, in a project that involves image recognition, each image must be labeled to identify objects within it. Here, labeling tool machine learning plays a significant role.
The Role of Labeling Tool Machine Learning in Business
Businesses today generate vast amounts of data. To capitalize on this data, they need robust solutions for its annotation. Here’s where labeling tool machine learning steps in, facilitating:
- Improved Accuracy: Automated labeling tools utilize ML algorithms to label data correctly, minimizing human error.
- Enhanced Efficiency: Such tools can process large volumes of data quickly, significantly reducing the time required for data preparation.
- Scalability: Businesses can scale their data annotation efforts without proportionately increasing their human resources.
- Cost-Effectiveness: By automating the annotation process, companies save on labor costs while still obtaining high-quality data.
Key Features of a Quality Labeling Tool
When considering a labeling tool for machine learning, it is essential to evaluate a range of features that ensure effectiveness and efficiency. Here are some characteristics to look for:
1. User-Friendly Interface
A good labeling tool should have an intuitive interface, making it easy for users to navigate and utilize its features without extensive training.
2. Support for Various Data Types
The tool should be capable of handling multiple types of data, including text, images, audio, and video, to accommodate diverse business needs.
3. Collaboration Features
In a team-oriented environment, the ability to collaborate seamlessly allows multiple users to annotate data concurrently, improving productivity.
4. Customizability
Organizations often have unique requirements. A labeling tool that allows for custom label sets and workflows can adapt to these needs effectively.
5. Integration Capabilities
Compatibility with existing systems and workflows is crucial. Look for a labeling tool that integrates with data pipelines and machine learning frameworks.
Benefits of Choosing KeyLabs AI
Among various options available in the market, KeyLabs AI stands out as a leading data annotation platform. Here’s why:
- Comprehensive Solutions: KeyLabs AI offers a suite of tools that cater to various aspects of data annotation, ensuring that users have everything they need in one place.
- Advanced Machine Learning Integration: Its platform is designed to leverage the latest in machine learning technology for enhanced annotation capabilities.
- Scalable Infrastructure: The platform can scale with your business, handling even the most extensive annotation tasks without compromising on performance.
- Expert Support: Users benefit from dedicated support from industry experts, ensuring they can maximize the platform’s potential.
Best Practices for Using Labeling Tools in Machine Learning
To optimize the use of labeling tools, consider the following best practices:
1. Define Clear Annotation Guidelines
Clearly defined guidelines help ensure consistency across annotations, which is crucial for training effective machine learning models.
2. Regularly Review and Audit Annotations
Regularly reviewing annotations for quality and accuracy can prevent errors that could compromise the model's performance.
3. Utilize Feedback Loops
Encourage annotators to provide feedback about the tool and the data. This can lead to improvements in both the tool and the annotation process.
4. Train Annotators Effectively
Investing in training for your team will pay off. Ensuring that annotators understand the tool and the context of the work enhances quality.
5. Start Small and Scale
Begin the tagging process with a pilot project to test the tool's effectiveness before scaling up to larger data sets.
Conclusion
The integration of labeling tool machine learning in business operations is no longer an option but a necessity. The quality of data annotation can significantly influence the outcomes of machine learning projects, and thus utilizing proficient tools like those offered by KeyLabs AI is essential. By investing in the best data annotation tools, businesses can ensure that they harness the full potential of their data, leading to informed decision-making and a competitive edge in their respective markets.
In a rapidly evolving digital landscape, embracing these innovative technologies will allow companies not only to keep pace with change but to thrive and lead in their industries.